Large language models are artificial intelligence tools that are trained on mass amounts of data and can understand and respond to user prompts. Current LLMs are known for their ability to generate text, but these models are now evolving to be able to create images and video.
The ability of LLMs to break down huge datasets offers public sector organizations many opportunities to enhance their decision making capabilities. Though LLMs hold immense potential, they still have issues, and experts say that a human-in-the-loop is necessary for these tools to be used effectively.
“We’ve gone from a search engine to an answer engine, but the analyst is also required. And so, we’re trying to use them as a partner to help inform and get through the massive amounts of data to help inform decision making,” Col. Michael Medgyessy, intelligence chief information officer for the U.S. Department of the Air Force, explained during a panel discussion at the Potomac Officers Club’s 5th Annual CIO Summit on Wednesday.
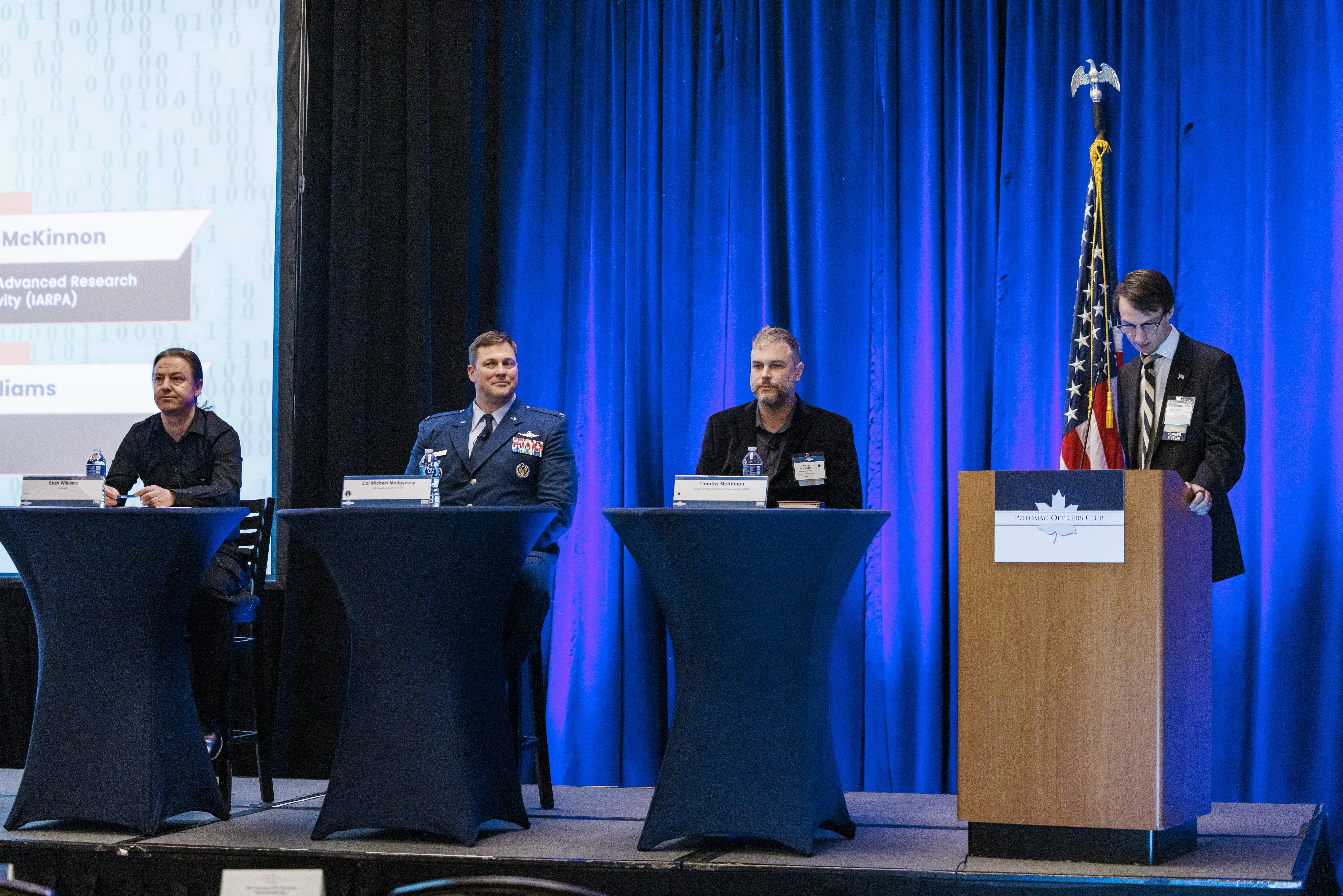
Sean Williams, founder and global CEO of AutogenAI, pointed out that because LLMs can access such wide amounts of information, there is a significant chance that their analysis, though done very quickly, may not represent the truth as accurately as a human could.
“For accuracy, we want to use that ability to read and then we want to apply human notions of, ‘what is truth, what is a trusted source?’” he said.
Some of this is a technical issue, but according to Williams, it is also a “philosophical problem about what we actually mean by ‘truth’” and how that concept can be matched with new AI technologies.
Despite these concerns, Timothy McKinnon, a program manager at the Intelligence Advanced Research Projects Activity, said the inferences made by LLMs should still be made available to users.
“What we need ultimately is a taxonomy or an understanding of all the different ways in which inferences can be good and bad,” he said.
Another challenge LLMs present is bias. McKinnon said that the assumption that it is possible to create unbiased models is flawed due to how LLMs function. Since these models draw from such large amounts of data, they often pick up information from sources that are biased themselves, he noted.
“I think that instead of trying to de-bias models, what we should be trying to do is trying to induce perspectives based on an interesting understanding of bias,” he said.
To do so, McKinnon recommended organizations “try to understand bias and use it to induce a set of diverse perspectives and play off of the analyst’s creativity.”
Medgyessy brought up data tagging – which he said offers an “opportunity for models to be actually really good because the data sets that it is reading are really good” – as a way to combat problems with accuracy.
“When you train something on [properly tagged data], it’s like sending it to school – not to the playground – to figure out what it’s like,” he added.
In the future, Medgyessy said that what he sees as the biggest threat in the LLM space is “how humans actually receive information and in their own decisions, being manipulated.”

The Potomac Officers Club’s next event, the 2024 5G Forum, will dive into how federal agencies are using modern network technologies to accomplish their missions. To learn more and register to attend the event, which will feature public and private sector 5G experts, click here.